Abstract
Detecting personalities in social media content is an important application of personality psychology. Most early studies apply a coherent piece of writing to personality detection, but today, the challenge is to identify dominant personality traits from a series of short, noisy social media posts. To this end, recent studies have attempted to individually encode the deep semantics of posts, often using attention-based methods, and then relate them, or directly assemble them into graph structures. However, due to the inherently disjointed and noisy nature of social media content, constructing meaningful connections remains challenging. While such methods rely on well-defined relationships between posts, effectively capturing these connections in fragmented and sparse content is non-trivial, particularly under limited supervision or noisy input. To tackle this, we draw inspiration from the scanning reading technique—commonly recommended for efficiently processing large volumes of information—and propose an index attention mechanism as a solution. This mechanism leverages prior psycholinguistic knowledge as an “index” to guide attention, thereby enabling more effective information fusion across scattered semantic signals. Building on this idea, we introduce the Index Attention Network (IAN)—a novel framework designed to infer personality labels by performing targeted information fusion over deep semantic representations of individual posts. Through a series of experiments, IAN achieved state-of-the-art performance on the Kaggle dataset and performance comparable to graph convolutional networks (GCN) on the Pandora dataset. Notably, IAN delivered an average improvement of 13% in terms of macro-F1 scores with the Kaggle dataset. The code for IAN is available at GitHub: https://github.com/Once2gain/IAN.
Data Availability Statement
The source code used in this study is publicly available on GitHub at the following link: https://github.com/Once2gain/IAN.
Funding
This work was supported by the Natural Science Foundation of Shandong Province under Grant ZR2020MF154.
Conflicts of Interest
The authors declare no conflicts of interest.
Ethical Approval and Consent to Participate
This study utilizes an anonymized public dataset, which is publicly available and does not contain any personally identifiable information. As the dataset is fully anonymized and used without the collection of personal data from individuals, ethical approval is not required for this research.
Cite This Article
APA Style
Tang, Q., Jiang, W., Pan, X., Lin, L., Zhu, J., Du, Y., & Sun, D. (2025). Using Psycholinguistic Clues to Index Deep Semantic Evidences: Personality Detection in Social Media Texts. Chinese Journal of Information Fusion, 2(2), 112–126. https://doi.org/10.62762/CJIF.2025.820998
Publisher's Note
IECE stays neutral with regard to jurisdictional claims in published maps and institutional affiliations.
Rights and permissions
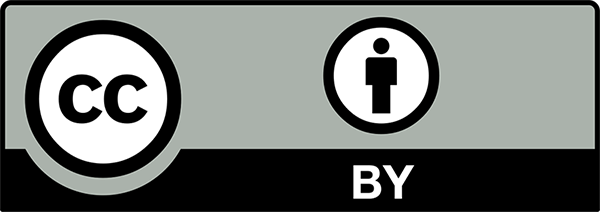
Copyright © 2025 by the Author(s). Published by Institute of Emerging and Computer Engineers. This article is an open access article distributed under the terms and conditions of the Creative Commons Attribution (CC BY) license (
https://creativecommons.org/licenses/by/4.0/), which permits use, sharing, adaptation, distribution and reproduction in any medium or format, as long as you give appropriate credit to the original author(s) and the source, provide a link to the Creative Commons licence, and indicate if changes were made.