Abstract
Due to the poor imaging quality of remote sensing images and the small size of targets, remote sensing small target detection has become a current research difficulty and hotspot. Recent years have seen many new algorithms. Remote sensing small target detection methods based on image super-resolution reconstruction have attracted many researchers due to their excellent performance. However, these algorithms still have problems such as weak feature extraction capability and insufficient feature fusion. Then, we propose Enhanced Feature based Small Target Detection Network in Remote Sensing Images (EFSOD), which includes a Edge Enhancement Super-Resolution Reconstruction Module (EESRM) and a Cross-Model Feature Fusion Module (CMFFM). EESRM enhances the recognizability of small target contours by fusing extracted edge features with the original features through residual connections, alleviating the constraints of feature blurring on detection performance. CMFFM achieves deep integration of the detailed features extracted by the EESRM network with the semantic features extracted by the target detection network, improving the model's sensitivity and accuracy in recognizing small targets in complex backgrounds. Additionally, considering the effects of blurring, noise, illumination changes, and atmospheric scattering on remote sensing images, a remote sensing image degradation simulation algorithm is proposed. This algorithm realistically simulates the generation process of low-resolution remote sensing images under natural conditions, providing more realistic training and testing data. The experimental results show that the proposed EFSOD significantly enhances the performance of small object detection in remote sensing.
Keywords
remote sensing images
super-resolution
small object detection
cross-model feature fusion
Data Availability Statement
Data will be made available on request.
Funding
This work was supported in part by the National Natural Science Foundation of China under Grant 62076165 and under Grant 62471317; in part by the Innovation Team Project of Department of Education of Guangdong Province under Grant 2020KCXTD004; in part by the Guangdong Provincial Key Laboratory under Grant 2023B1212060076.
Conflicts of Interest
The authors declare no conflicts of interest.
Ethical Approval and Consent to Participate
Not applicable.
Cite This Article
APA Style
Yi, J., Liu, Y., Li, Y., & Xie, W. (2025). EFSOD: Enhanced Feature based Small Object Detection Network in Remote Sensing Images. Chinese Journal of Information Fusion, 2(2), 127–143. https://doi.org/10.62762/CJIF.2025.845143
Publisher's Note
IECE stays neutral with regard to jurisdictional claims in published maps and institutional affiliations.
Rights and permissions
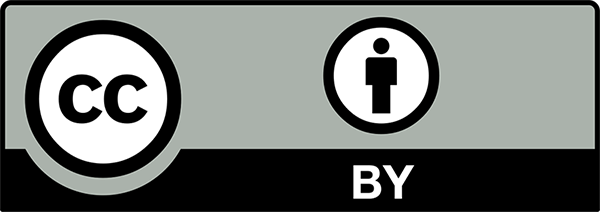
Copyright © 2025 by the Author(s). Published by Institute of Emerging and Computer Engineers. This article is an open access article distributed under the terms and conditions of the Creative Commons Attribution (CC BY) license (
https://creativecommons.org/licenses/by/4.0/), which permits use, sharing, adaptation, distribution and reproduction in any medium or format, as long as you give appropriate credit to the original author(s) and the source, provide a link to the Creative Commons licence, and indicate if changes were made.