Abstract
Food security is crucial for human survival and national economic development, but frequent meteorological disasters have caused great harm to agricultural production. Therefore, it is very important and meaningful to study how to quickly and accurately predict the loss rate of disasters. Only based on historical loss sequence, the time series prediction method can effectively predict future loss. Therefore, this paper first briefly describes the main means of time series prediction, namely statistical methods and machine learning algorithms. Secondly, the commonly used machine learning algorithms for disaster loss time series prediction, and its application cases and existing problems, were introduced in detail. To address the issue of small sample sizes for loss predication, data augmentation techniques can be used; To address the issue of data non-stationarity, Empirical Mode Decomposition (EMD) can be used to decompose the original sequence into relatively stationary sub-sequences. In addition, exploratory solutions have been proposed, such as ensemble learning strategies for multiple machine learners, and combining machine learning algorithms with optimization algorithms, strong prediction strategies, or attention mechanisms. Finally, a summary solution for conventional disaster damage prediction was proposed.
Data Availability Statement
Not applicable.
Funding
This work was supported without any funding.
Conflicts of Interest
Feng Fang, Jingjing Lin, Peixuan Chen, Pengcheng Huang, Xing Wang, Yulong Ma, Liwei Liu, and Xiaowei Wang are employees of the Lanzhou Regional Climate Center, Lanzhou 730020, China. Jing Wang is an employee of the Lanzhou Institute of Arid Meteorology, Lanzhou 730020, China.
Ethical Approval and Consent to Participate
Not applicable.
Cite This Article
APA Style
Fang, F., Lin, J., Wang, J., Chen, P., Huang, P., Wang, X., Ma, Y., Liu, L., Wang, D., & Wang, X. (2024). Research Progress on Time Series Prediction of Agricultural Disaster Risk: A Mini-review. Agricultural Science and Food Processing, 2(1), 1–11. https://doi.org/10.62762/ASFP.2024.610643
Publisher's Note
IECE stays neutral with regard to jurisdictional claims in published maps and institutional affiliations.
Rights and permissions
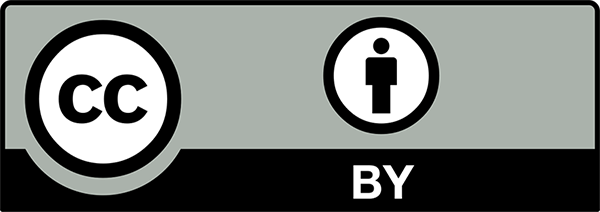
Copyright © 2024 by the Author(s). Published by Institute of Emerging and Computer Engineers. This article is an open access article distributed under the terms and conditions of the Creative Commons Attribution (CC BY) license (
https://creativecommons.org/licenses/by/4.0/), which permits use, sharing, adaptation, distribution and reproduction in any medium or format, as long as you give appropriate credit to the original author(s) and the source, provide a link to the Creative Commons licence, and indicate if changes were made.