IECE Transactions on Emerging Topics in Artificial Intelligence | Volume 1, Issue 1: 71-83, 2024 | DOI:10.62762/TETAI.2024.305743
Abstract
Recently, the primary reason for blindness in adults has been diabetic retinopathy (DR) disease. Therefore, there is an increasing demand for a real-time efficient classification and detection system for diabetic retinopathy (DR) to overcome fast-growing disease (DR). We introduced a novel deep hybrid model for auto-mated diabetic retinopathy (DR) disease recognition and classification. Our model leverages the power of CNN architectures: Inception V3 and VGG16 models by combining their strengths to cater to exact requirements. VGG16 model efficiently captures fine features and wide-ranging features such as textures and edges, crucial for classifying initial signs of DR. Similarly, Inception... More >
Graphical Abstract
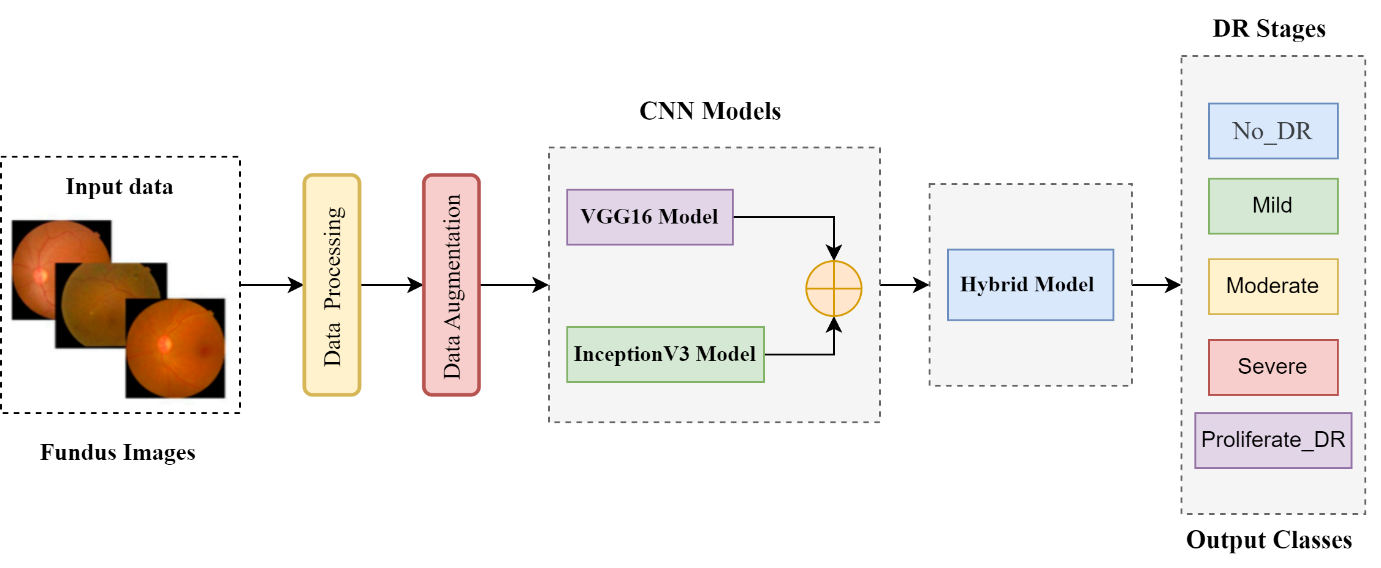