IECE Transactions on Emerging Topics in Artificial Intelligence | Volume 2, Issue 1: 43-56, 2025 | DOI: 10.62762/TETAI.2025.222799
Abstract
Public health surveillance is crucial for early disease detection, outbreak prediction, and epidemic response. However, traditional surveillance systems primarily rely on structured clinical data, limiting their capacity to capture emerging health threats from diverse and unstructured sources. This study explores the integration of Natural Language Processing (NLP) and Artificial Intelligence (AI) to automate disease surveillance by analyzing unstructured data, including electronic health records (EHRs), social media posts, news reports, and online health forums. Leveraging state-of-the-art NLP techniques—such as transformer-based language models, named entity recognition (NER), sentiment... More >
Graphical Abstract
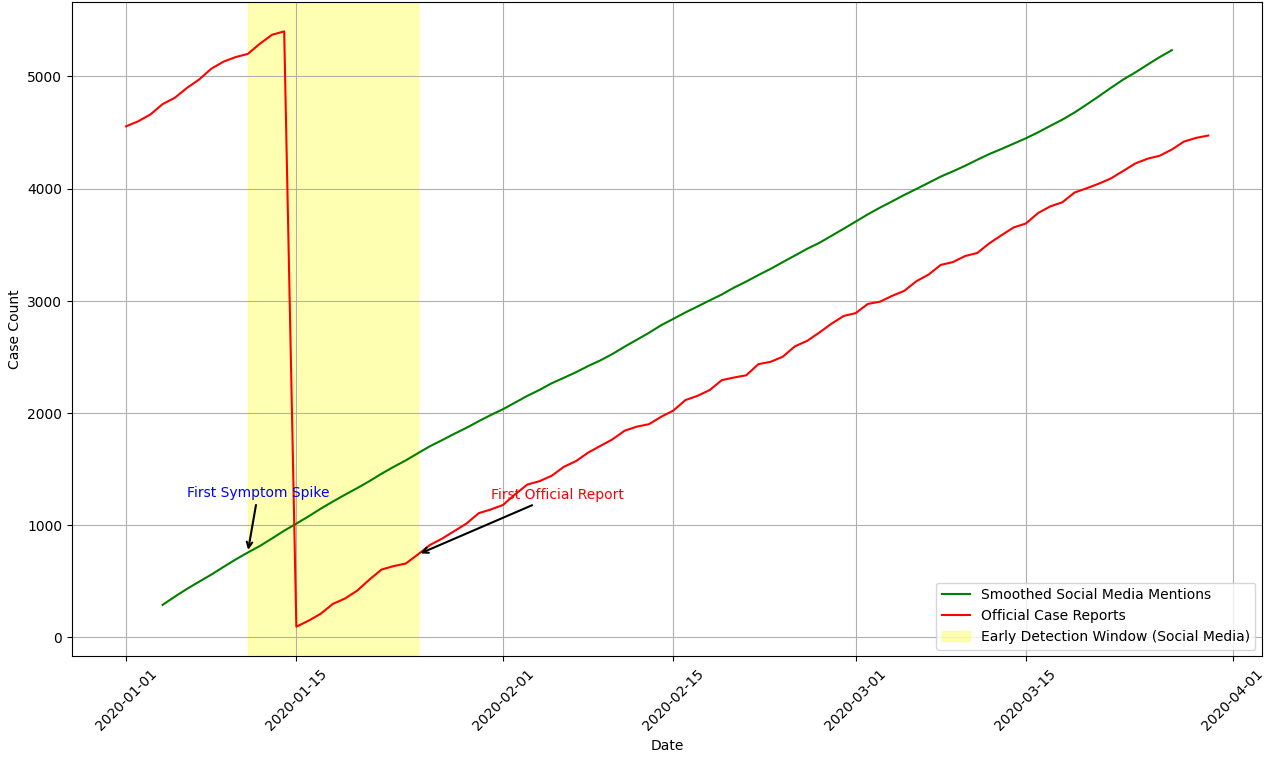