IECE Transactions on Emerging Topics in Artificial Intelligence | Volume 1, Issue 1: 58-70, 2024 | DOI:10.62762/TETAI.2024.628205
Abstract
This paper proposes an improved video action recognition method, primarily consisting of three key components. Firstly, in the data preprocessing stage, we developed multi-temporal scale video frame extraction and multi-spatial scale video cropping techniques to enhance content information and standardize input formats. Secondly, we propose a lightweight Inception-3D networks (LI3D) network structure for spatio-temporal feature extraction and design a soft-association feature aggregation module to improve the recognition accuracy of key actions in videos. Lastly, we employ a bidirectional LSTM network to contextualize the feature sequences extracted by LI3D, enhancing the representation capa... More >
Graphical Abstract
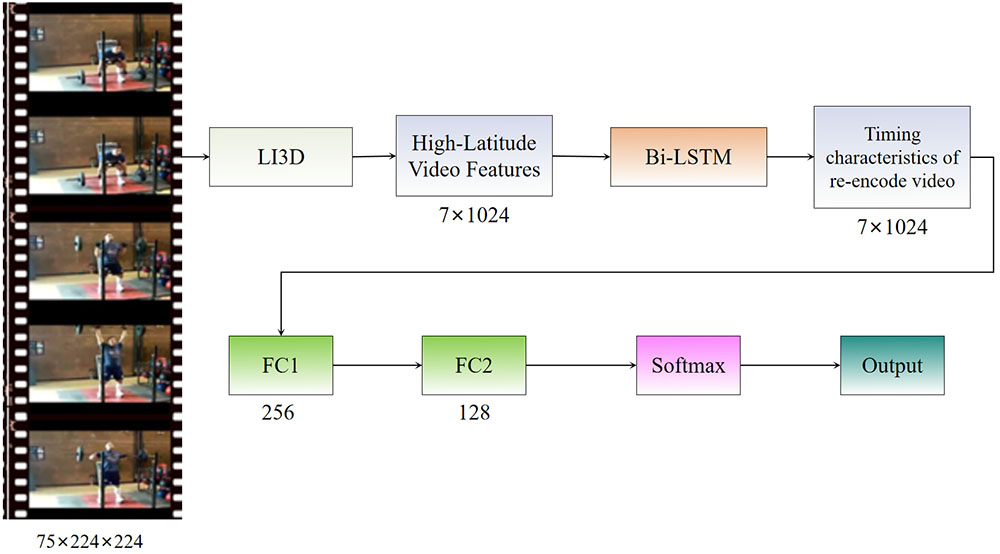