IECE Transactions on Intelligent Systematics | Volume 2, Issue 1: 1-13, 2024 | DOI:10.62762/TIS.2025.367320
Abstract
In the healthcare sector, the application of deep learning technologies has revolutionized data analysis and disease forecasting. This is particularly evident in the field of diabetes, where the deep analysis of Electronic Health Records (EHR) has unlocked new opportunities for early detection and effective intervention strategies. Our research presents an innovative model that synergizes the capabilities of Bidirectional Long Short-Term Memory Networks-Conditional Random Field (BiLSTM-CRF) with a fusion of XGBoost and Logistic Regression. This model is designed to enhance the accuracy of diabetes risk prediction by conducting an in-depth analysis of electronic medical records data. The fir... More >
Graphical Abstract
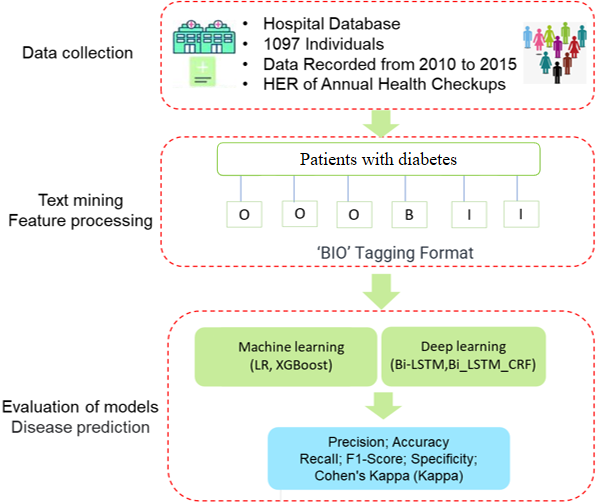