IECE Transactions on Intelligent Systematics | Volume 2, Issue 1: 14-26, 2024 | DOI:10.62762/TIS.2025.613103
Abstract
Indoor scene recognition poses considerable hurdles, especially in cluttered and visually analogous settings. Although several current recognition systems perform well in outside settings, there is a distinct necessity for enhanced precision in inside scene detection, particularly for robotics and automation applications. This research presents a revolutionary deep Convolutional Neural Network (CNN) model tailored with bespoke parameters to improve indoor picture comprehension. Our proprietary dataset consists of seven unique interior scene types, and our deep CNN model is trained to attain excellent accuracy in classification tasks. The model exhibited exceptional performance, achieving a t... More >
Graphical Abstract
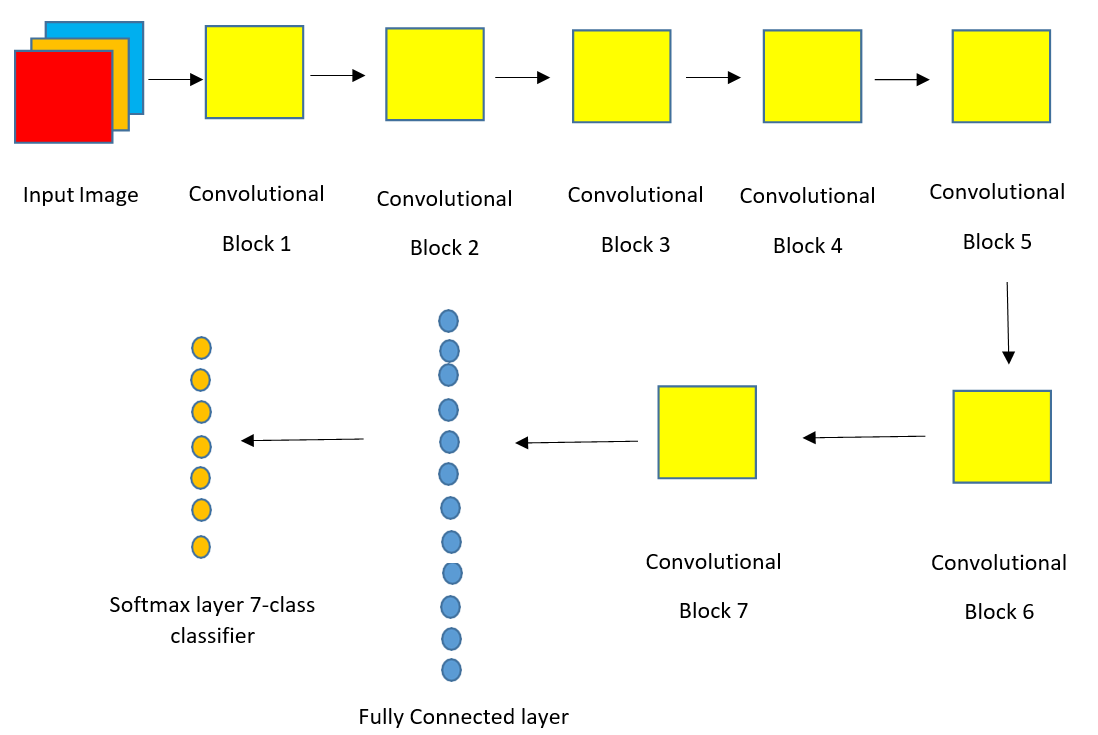