IECE Transactions on Sensing, Communication, and Control | Volume 2, Issue 1: 36-47, 2025 | DOI: 10.62762/TSCC.2025.378503
Abstract
Osteoarthritis (OA) is a degenerative joint disease that primarily affects the knee, causing cartilage deterioration and discomfort. Early diagnosis is crucial for effective management, as it can slow disease progression and improve the quality of life. This study proposes a deep learning approach to automatically classify knee OA severity from X-ray images using Convolutional Neural Networks (CNNs) and the VGG16 model. The models were trained on a dataset of knee X-ray images, and performance was evaluated using accuracy, precision, recall, and F1-score. The proposed CNNs model achieved 99% training accuracy and 80% testing accuracy after 50 epochs, while the VGG16 model, after fine-tuning... More >
Graphical Abstract
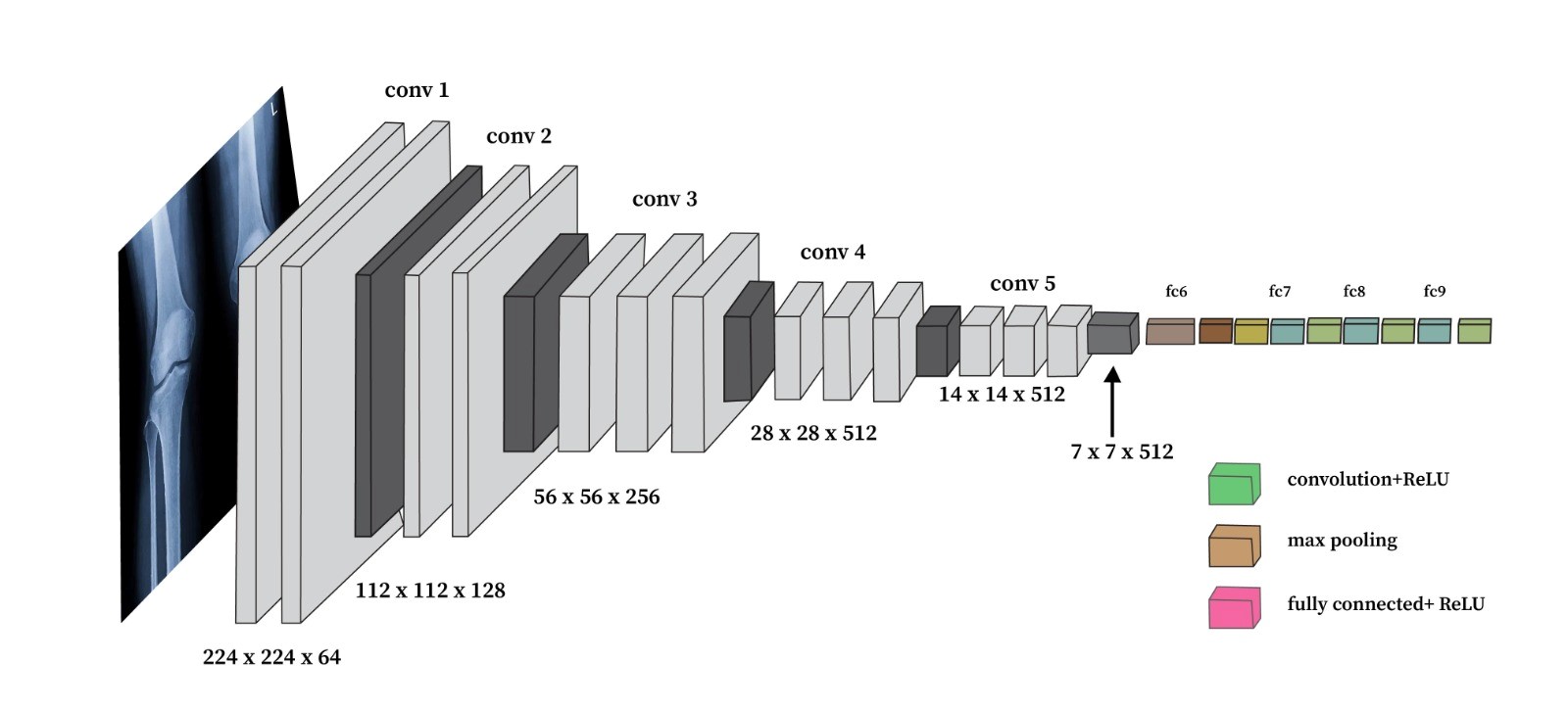