IECE Transactions on Neural Computing | Volume 1, Issue 1: 44-54, 2025 | DOI: 10.62762/TNC.2025.737491
Abstract
The rapid evolution of digital threats is a major hurdle to the security of vital infrastructure, driving the need for advanced cybersecurity methods like those based on the use of new technologies. This research seeks to assess the use of neural networks in cybersecurity and especially the role of these technologies in proactive risk management and threat intelligence. Neural networks, mainly deep learning models, had excellent success in detecting, analyzing, and mitigating all cyber threats with no time delay. Through the integration of sophisticated components such as pattern recognition, anomaly detection, and predictive analytics, these models improve threat detection accuracy while mi... More >
Graphical Abstract
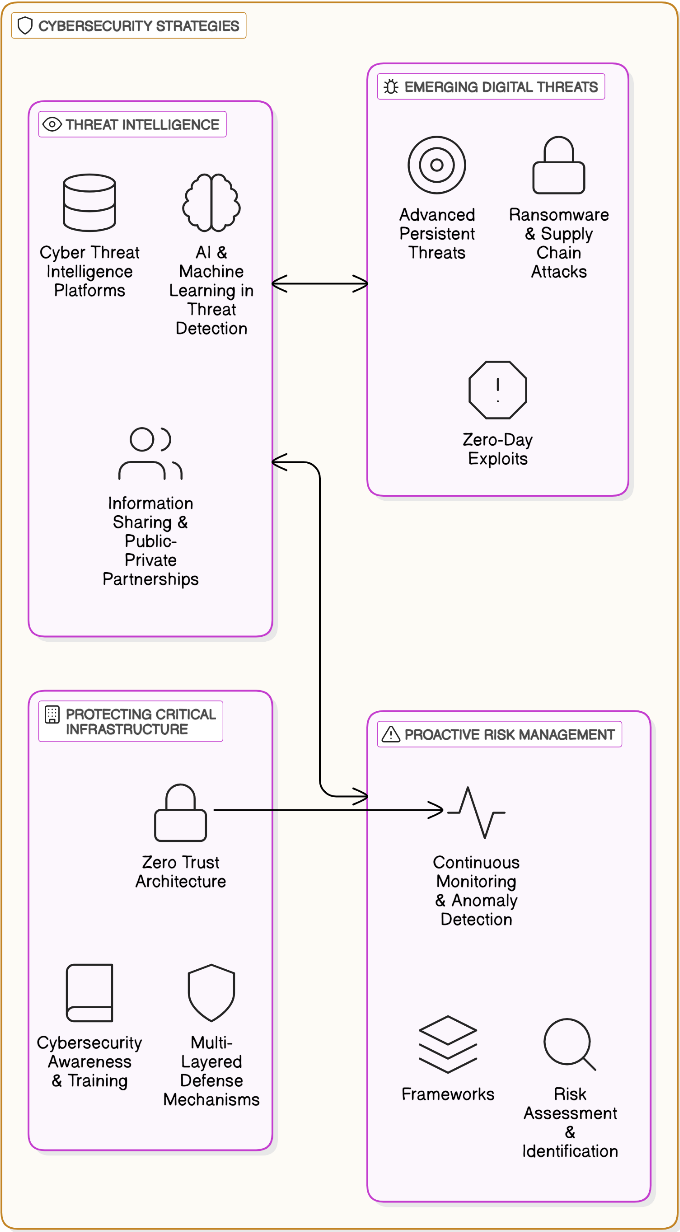