Abstract
The global healthcare systems have faced unprecedented challenges due to the COVID-19 pandemic, necessitating innovative neural computing solutions to inform critical decision-making. In this study, we introduce a neural-inspired machine learning framework to predict COVID-19 mortality risk, utilizing a dataset comprising over one million records. We developed and evaluated a suite of advanced models—Decision Tree, Random Forest, Logistic Regression, Support Vector Machine, Gradient Boost Classifier, and a neural ensemble-based Voting Classifier—to analyze the influence of demographics, symptoms, and preexisting conditions on mortality predictions. Through meticulous feature engineering and data preprocessing, our approach yielded profound insights, with the Voting Classifier achieving an exceptional 93% accuracy on test data, outperforming Random Forest and Logistic Regression (both at 91%). Key risk factors identified include age and preexisting conditions, complemented by nuanced patterns linked to symptoms and socioeconomic-demographic factors. Model robustness was rigorously validated using F1-score and ROC curves, affirming its reliability and generalization capacity. The Voting Classifier’s neural ensemble design, integrating diverse model outputs, exemplifies the power of neural computing principles in processing complex health data. This framework not only enhances predictive accuracy but also provides actionable insights for public health, enabling optimized resource allocation, prioritized care for high-risk patients, and improved survival outcomes. Beyond elucidating COVID-19 mortality dynamics, this research underscores the transformative potential of neural computing in tackling global health crises, establishing a robust foundation for data-driven strategies in future challenges.
Keywords
COVID-19
health care analytics
predictive modeling mortality risk
Data Availability Statement
Data will be made available on request.
Funding
This work was supported without any funding.
Conflicts of Interest
The authors declare no conflicts of interest.
Ethical Approval and Consent to Participate
This work uses publicly available, anonymized Mexico General Directorate of Epidemiology COVID-19 Open Data from the General Directorate of Epidemiology under the Ministry of Health of Mexico. All data were fully de-identified prior to use. Therefore, ethical approval and informed consent were not required, in accordance with institutional guidelines and applicable regulations.
Cite This Article
APA Style
Hasnain, M. A., Abbas, T., Janjua, J. I., & Khan, S. (2025). Uncovering COVID-19 Death Risk for Life on the Line with Machine Learning Precision. IECE Transactions on Neural Computing, 1(1), 30–43. https://doi.org/10.62762/TNC.2025.507897
Publisher's Note
IECE stays neutral with regard to jurisdictional claims in published maps and institutional affiliations.
Rights and permissions
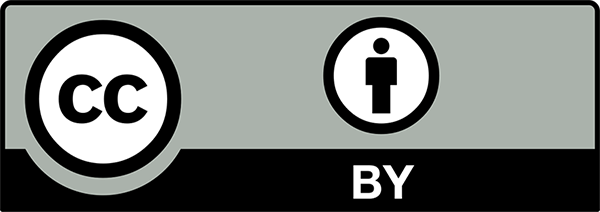
Copyright © 2025 by the Author(s). Published by Institute of Emerging and Computer Engineers. This article is an open access article distributed under the terms and conditions of the Creative Commons Attribution (CC BY) license (
https://creativecommons.org/licenses/by/4.0/), which permits use, sharing, adaptation, distribution and reproduction in any medium or format, as long as you give appropriate credit to the original author(s) and the source, provide a link to the Creative Commons licence, and indicate if changes were made.