Abstract
Neural network-enhanced machine learning is revolutionizing cybersecurity by enabling real-time detection of anomalous activities and proactive prevention of unauthorized access in large-scale networks. Traditional security measures often prove ineffectual in the face of the fast-developing threats, as they depend on unchanging rules and signature detections, which can be bypassed by the advanced cyber adversaries. In contrast, neural networks apply deep learning techniques to several data sets including user behavior, network traffic, and system activity, which helps them to spot small irregularities that may mean a potential threat. By feed-forwarding new information on the high-quality training sets, the AI-based models got the better of the specific task and entrenched themselves in the industry, thus allowing them to speed detection, reduce false positives, and automate threat-responsiveness for the proper solution of problems .In addition, neural networks also comprise the crucial aspects of the utilization of adaptive access control, the deepening of identity verification processes, as well as eliminating the risk of insider threats and zero-day vulnerabilities. However, neural networks in cybersecurity can also pose challenges, such as adversarial attacks, interpretability issues of the model, and concerns regarding data privacy. Solving these problems using adversarial training, explainable AI (XAI), and ethical AI governance is required to ensure that the potential of neural networks can be used to the greatest extent in the context of cybersecurity. With the changing paradigm from reactive to proactive security measures, organizations are capitalizing on the robust and intelligent resource that neural networks are in combating increasing cyber threats and restoring the security of extensive digital infrastructures.
Keywords
neural networks
cybersecurity
anomaly detection
machine learning
unauthorized access prevention
Data Availability Statement
Data will be made available on request.
Funding
This work was supported without any funding.
Conflicts of Interest
The authors declare no conflicts of interest.
Ethical Approval and Consent to Participate
Not applicable.
Cite This Article
APA Style
Batool, F., & Hassnain, S. I. (2025). Neural Network-Enhanced Machine Learning Applications in Cybersecurity for Real-Time Detection of Anomalous Activities and Prevention of Unauthorized Access in Large-Scale Networks. IECE Transactions on Neural Computing, 1(1), 55–64. https://doi.org/10.62762/TNC.2025.920886
Publisher's Note
IECE stays neutral with regard to jurisdictional claims in published maps and institutional affiliations.
Rights and permissions
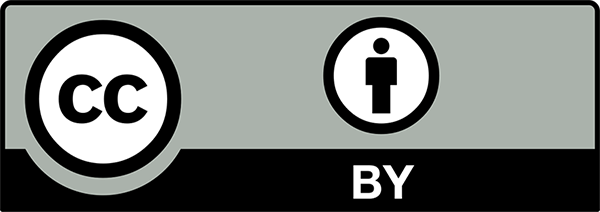
Copyright © 2025 by the Author(s). Published by Institute of Emerging and Computer Engineers. This article is an open access article distributed under the terms and conditions of the Creative Commons Attribution (CC BY) license (
https://creativecommons.org/licenses/by/4.0/), which permits use, sharing, adaptation, distribution and reproduction in any medium or format, as long as you give appropriate credit to the original author(s) and the source, provide a link to the Creative Commons licence, and indicate if changes were made.