Abstract
Predicting personality traits automatically has emerged as a challenging problem in computer vision. This paper introduces an innovative multimodal feature learning framework for personality analysis in short video clips. For visual processing, we construct a facial graph and design a Geo-based two-stream network incorporating an attention mechanism, leveraging both Graph Convolutional Networks (GCN) and Convolutional Neural Networks (CNN) to capture static facial expressions. Additionally, ResNet18 and VGGFace networks are employed to extract global scene and facial appearance features at the frame level. To capture dynamic temporal information, we integrate a BiGRU with a temporal attention module for extracting salient frame representations. To further enhance the model’s robustness, we incorporate the VGGish CNN for audio-based features and XLM-Roberta for text-based features. Finally, a multimodal channel attention mechanism is introduced to integrate different modalities, and a Multi-Layer Perceptron (MLP) regression model is utilized to predict personality traits. Experimental results confirm that our proposed framework surpasses existing state-of-the-art approaches in performance.
Keywords
personality prediction
facial graph
graph convolutional network(GCN)
convolutional neural network(CNN)
attention mechanism
geometric and appearance features
Data Availability Statement
Data will be made available on request.
Funding
This work was supported without any funding.
Conflicts of Interest
Chengwei Ye is an employee of Homesite Group Inc, GA 30043, United States and Huanzhen Zhang is an employee of Payment Department, Chewy Inc, MA 02210, United States.
Ethical Approval and Consent to Participate
Not applicable.
Cite This Article
APA Style
Wang, K., Ye, C., Zhang, H., Xu, L., & Liu, S. (2025). Graph-Driven Multimodal Feature Learning Framework for Apparent Personality Assessment. IECE Transactions on Emerging Topics in Artificial Intelligence, 2(2), 57–67. https://doi.org/10.62762/TETAI.2025.279350
Publisher's Note
IECE stays neutral with regard to jurisdictional claims in published maps and institutional affiliations.
Rights and permissions
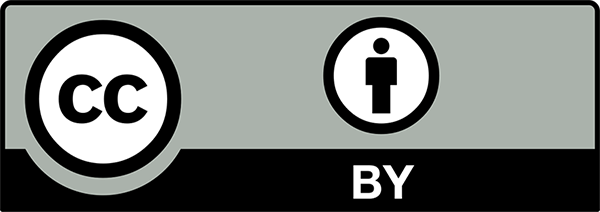
Copyright © 2025 by the Author(s). Published by Institute of Emerging and Computer Engineers. This article is an open access article distributed under the terms and conditions of the Creative Commons Attribution (CC BY) license (
https://creativecommons.org/licenses/by/4.0/), which permits use, sharing, adaptation, distribution and reproduction in any medium or format, as long as you give appropriate credit to the original author(s) and the source, provide a link to the Creative Commons licence, and indicate if changes were made.