Abstract
Few-shot learning aims to recognize new-class items under the circumstances with a few labeled support samples. However, many methods may suffer from poor guidance of limited new-class samples that are not suitable for being regarded as class centers. Recent works use word embedding to enrich the new-class distribution message but only use simple mapping between visual and semantic features during training. To solve the aforementioned problems, we propose a method that constructs a class relation graph by semantic meaning as guidance for feature extraction and fusion, to help the learning of the second-order relation information, with a light training request. In addition, we introduce two ways to generate pseudo prototypes for augmentation to resolve the lack of representation due to limited samples in novel classes: 1) A Generation Module(GM) that trains a small structure to generate visual features by using word embedding; 2) A Relation Module(RM) for training-free scenario that uses class relations in semantics to generate visual features. Extensive experiments on benchmarks including miniImageNet, CIFAR-FS and FC-100 prove that our method achieves state-of-the-art results.
Data Availability Statement
Data will be made available on request.
Funding
This work was supported by National Natural Science Foundation of China under Grant U20B2075.
Conflicts of Interest
Zijing Liu and Chenggang Wang are employees of the No.10th Research Institute, China Electronics Technology Group Corporation, Chengdu 610036, China.
Ethical Approval and Consent to Participate
Not applicable.
Cite This Article
APA Style
Liu, Z., & Wang, C. (2025). A Few-shot Learning Method Using Relation Graph. Chinese Journal of Information Fusion, 2(1), 70–78. https://doi.org/10.62762/CJIF.2025.146072
Publisher's Note
IECE stays neutral with regard to jurisdictional claims in published maps and institutional affiliations.
Rights and permissions
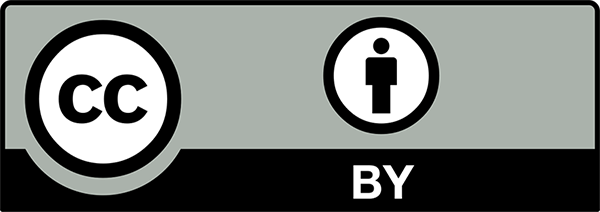
Copyright © 2025 by the Author(s). Published by Institute of Emerging and Computer Engineers. This article is an open access article distributed under the terms and conditions of the Creative Commons Attribution (CC BY) license (
https://creativecommons.org/licenses/by/4.0/), which permits use, sharing, adaptation, distribution and reproduction in any medium or format, as long as you give appropriate credit to the original author(s) and the source, provide a link to the Creative Commons licence, and indicate if changes were made.