Abstract
In the context of neural network-based radar feature extraction and detection methods, single-feature detection approaches exhibit limited capability in distinguishing targets from background in complex environments such as sea clutter. To address this, a Multi-Feature Extraction Network and Graph Fusion Detection Network (MFEn-GFDn) method is proposed, leveraging feature complementarity and enhanced information utilization. MFEn extracts features from various time-frequency maps of radar signals to construct Multi-Feature Graph Data (MFG) for multi-feature graphical representation. Subsequently, GFDn performs fusion detection on MFG containing multi-feature information. By expanding the feature dimension, detection performance is further improved. Experimental results on dataset composed of real measured IPIX data demonstrate that MFEn-GFDn detection probability is approximately 8% higher than that of the Dual-Channel Convolutional Neural Network (DCCNN). Additionally, MFEn-GFDn enhances detection performance by expanding the feature dimension, particularly in environments lacking corresponding training samples.
Keywords
radar target detection
feature fusion
graph data
graph fusion detection network
Data Availability Statement
Data will be made available on request.
Funding
This work was supported by National Natural Science Foundation of China under Grant 62222120 and Natural Science Foundation of Shandong under Grant ZR2024JQ003.
Conflicts of Interest
The authors declare no conflicts of interest.
Ethical Approval and Consent to Participate
Not applicable.
Cite This Article
APA Style
Su, N., Chen, X., Guan, J., Wang, X., Zhou, L., Wang, J., & Wang, H. (2025). Radar Multi-Feature Graph Representation and Graph Network Fusion Target Detection Methods. Chinese Journal of Information Fusion, 2(1), 59–69. https://doi.org/10.62762/CJIF.2025.413277
Publisher's Note
IECE stays neutral with regard to jurisdictional claims in published maps and institutional affiliations.
Rights and permissions
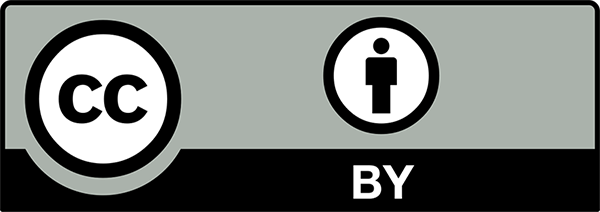
Copyright © 2025 by the Author(s). Published by Institute of Emerging and Computer Engineers. This article is an open access article distributed under the terms and conditions of the Creative Commons Attribution (CC BY) license (
https://creativecommons.org/licenses/by/4.0/), which permits use, sharing, adaptation, distribution and reproduction in any medium or format, as long as you give appropriate credit to the original author(s) and the source, provide a link to the Creative Commons licence, and indicate if changes were made.