Abstract
This study employs Response Surface Methodology (RSM) to model and optimize earthquake-induced ground movements in gravelly geohazard-prone environments. RSM efficiently evaluates the interactions of seismic parameters, including soil type, fault distance, and peak ground acceleration (PGA), reducing computational and experimental efforts. A dataset of 234 entries encompassing 11 seismic and soil stress variables was curated and analyzed, yielding a high-precision predictive model with an R² of 0.9997. The resulting closed-form equation facilitates accurate risk assessment, structural safety optimization, and seismic resilience planning. By identifying critical thresholds and nonlinear relationships, RSM supports cost-effective mitigation strategies, infrastructure design, and retrofitting in earthquake-prone regions.
Keywords
earthquake
ground movement
geohazard
seismic activity
response surface methodology (RSM)
liquefaction potential
Data Availability Statement
Data will be made available on request.
Funding
This work was supported without any funding.
Conflicts of Interest
The authors declare no conflicts of interest.
Ethical Approval and Consent to Participate
Not applicable.
Cite This Article
APA Style
Onyelowe, K. C., Kontoni, D. N., Onyelowe, F. K. C., Kamchoom, V., Hanandeh, S., Ebid, A. M., Ulloa, N., Moghal, A. A. B., & Vishnupriyan, M. (2025). Forecasting Earthquake-induced Ground Movement under Seismic Activity Using Response Surface. Sustainable Intelligent Infrastructure, 1(1), 4–18. https://doi.org/10.62762/SII.2025.846883
Publisher's Note
IECE stays neutral with regard to jurisdictional claims in published maps and institutional affiliations.
Rights and permissions
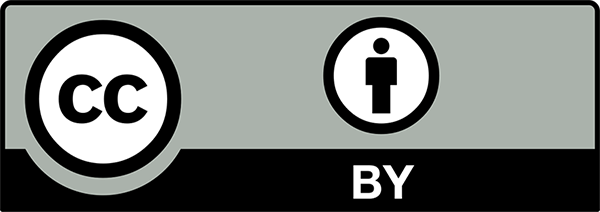
Copyright © 2025 by the Author(s). Published by Institute of Emerging and Computer Engineers. This article is an open access article distributed under the terms and conditions of the Creative Commons Attribution (CC BY) license (
https://creativecommons.org/licenses/by/4.0/), which permits use, sharing, adaptation, distribution and reproduction in any medium or format, as long as you give appropriate credit to the original author(s) and the source, provide a link to the Creative Commons licence, and indicate if changes were made.