Abstract
This paper introduces ViTDroid, a novel hybrid model that combines Vision Transformers (ViTs) and recurrent neural networks (RNNs) to enhance Android and IoT malware detection. ViTDroid addresses critical challenges by leveraging ViTs to capture global spatial dependencies and RNNs (LSTM and GRU) to model temporal patterns, enabling comprehensive analysis of complex malware behaviors. Additionally, the model integrates explainability tools, such as LIME and SHAP, to enhance transparency and trustworthiness, essential for real-world cybersecurity applications. The study evaluates ViTDroid's performance against conventional models, including RNN, LSTM, and GRU, using accuracy, precision, recall, and F1 score as evaluation metrics. Results demonstrate that ViTDroid achieves superior performance with an accuracy of 99.1% for Android malware and 98% for IoT malware. Precision and recall values reach 0.99 and 0.98, respectively, for Android, and 0.97 and 0.98 for IoT, with F1 scores of 0.99 for Android and 0.97 for IoT. These findings underscore ViTDroid's potential as a robust, efficient, and explainable solution to combat evolving threats in mobile and IoT ecosystems, paving the way for future advancements in malware detection systems.
Data Availability Statement
Data will be made available on request.
Funding
This work was supported without any funding.
Conflicts of Interest
The authors declare no conflicts of interest.
Ethical Approval and Consent to Participate
Not applicable.
Cite This Article
APA Style
Khan, U. I., Zeb, A., Rahman, T., Khan, F. M., Haider, Z. A., & Bilal, H. (2025). ViTDroid and Hybrid Models for Effective Android and IoT Malware Detection. IECE Transactions on Advanced Computing and Systems, 1(1), 32–47. https://doi.org/10.62762/TACS.2024.521915
Publisher's Note
IECE stays neutral with regard to jurisdictional claims in published maps and institutional affiliations.
Rights and permissions
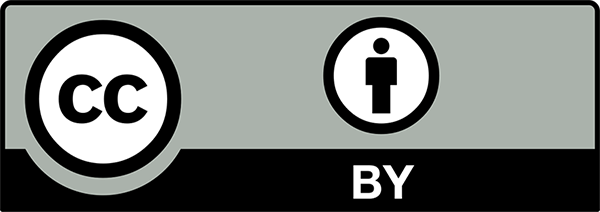
Copyright © 2025 by the Author(s). Published by Institute of Emerging and Computer Engineers. This article is an open access article distributed under the terms and conditions of the Creative Commons Attribution (CC BY) license (
https://creativecommons.org/licenses/by/4.0/), which permits use, sharing, adaptation, distribution and reproduction in any medium or format, as long as you give appropriate credit to the original author(s) and the source, provide a link to the Creative Commons licence, and indicate if changes were made.